Data-driven decision making - do’s and don'ts when designing the business process
With a background in engineering and a keen interest in mathematics, I am absolutely thrilled by the infrastructure that is growing around collecting, analyzing and acting on data in businesses. There is a real hype around data-driven decision making (DDDM) and ‘turning data into action’. However, throughout this hype, I have started to develop a growing concern for how businesses handle data and I question whether or not everything really can and should be converted into figures.
What is so great about data?
To start from the beginning, what is great about data is that it is objective in the sense that it is externally verifiable, as opposed to internally non-verifiable factors, such as one’s feelings or experience. Studies have also shown that business that use data in their decision making (as opposed to feelings and experience) increase their productivity and revenue. This is of course great news for businesses who want to grow and for data analytics professionals. Another great piece of news is that the costs associated with collecting data is falling and it is more easily accessible than before.
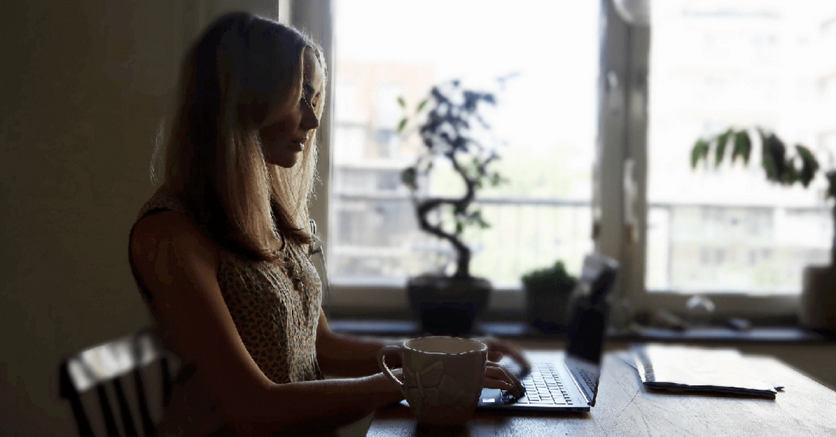
What is difficult about data?
When it comes to handling data, it is common for businesses to miss-use the data that they have gathered or to end up not using it at all. On that note, companies only use 50 % of the data they collect, according to a recent study. However, if we do use the data, there are a number of ways throughout the process in which we inflict errors and biases in the data. First off, we tend to ‘cherry pick’ to collect data within our comfort zone. It is really no surprise to anyone that businesses gather data that is quick and cost-effective to collect, but by doing so, we are immediately exposing the data for selection bias.
When analyzing data, we continue ‘cherry picking’ among the data that is collected and we sometimes end up using data that fits our predetermined view. This is something we do both consciously and subconsciously. We are also great at associating, creating and seeing patterns in the data that are not there. The analytical and critical step in the data extraction process is probably the most difficult, and most overlooked, part of it all. While there are numerous, comprehensive and good data analytics software and tools out there, the analysis often takes place in Excel to some extent. It is important to know its limitations and its invitations to formula errors, both big and small. Depending on one’s Excel skills, it can be really difficult to track errors and a big risk with Excel is that an error can be long-lived - getting passed on to the next calculation template. There is also a huge risk of generalizing too much and too often. For example, in data conversions, comparisons and when merging data time series.
What to think about when designing the business process
Here are some concrete actions to take and concepts to look up if you want to get better at data-driven decision making:
Business processes
- Create continuous and standardized report flows, be transparent with the data in the company, hire data analytics professionals and remember that outsourcing the whole process of data gathering and analyzing, or parts of it, or buying data from third parties, are possibilities that are worthy to consider.
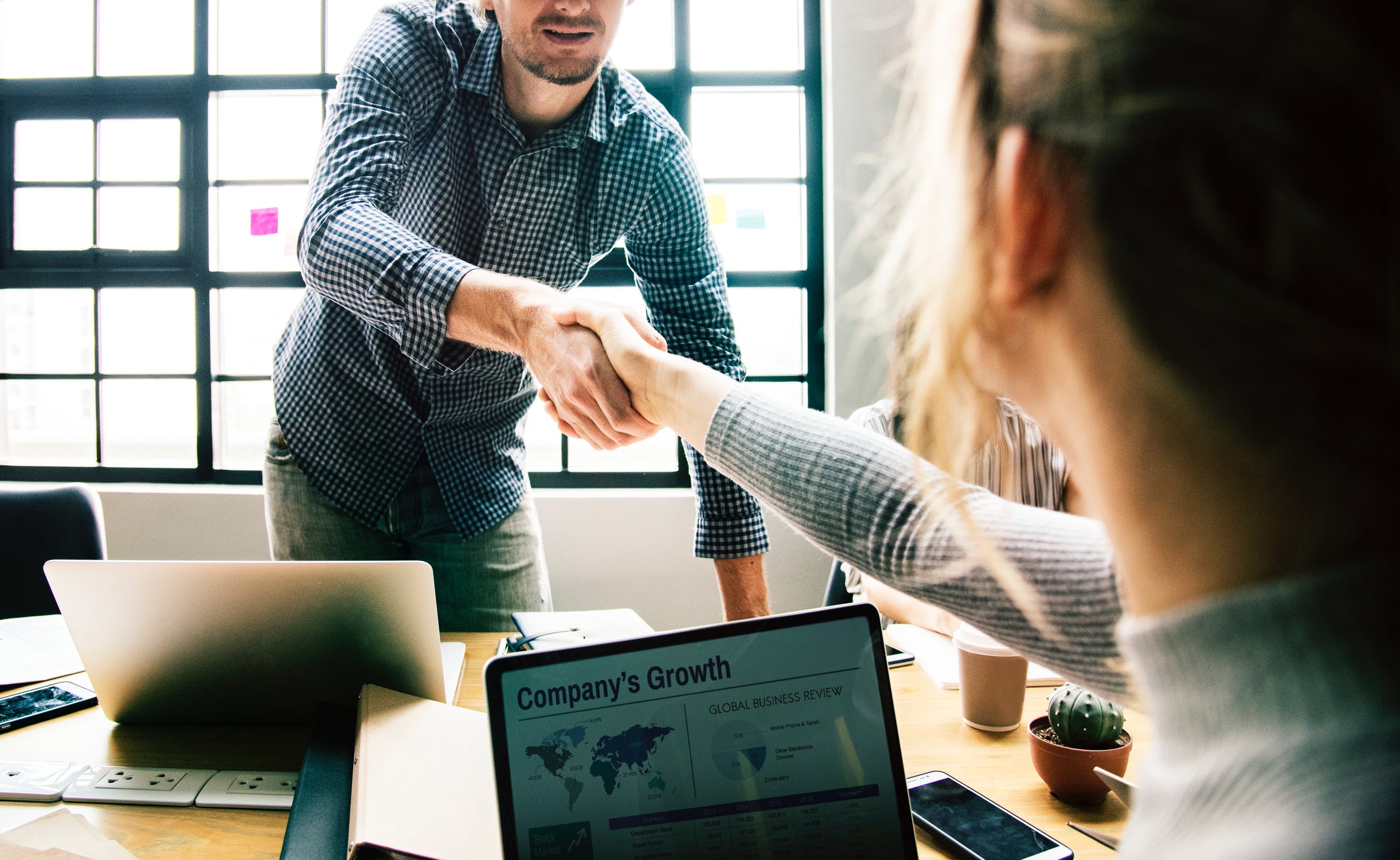
KPI:s
- Define clear, firm-wide KPI:s and make sure that everyone is measuring the same thing - the more specific the better. In order to do this, think like this: “We collect and measure X and Y in order to answer question Z”.
- Do not be afraid of going out of your comfort zone when collecting data. Even if it is more of a hassle to measure it, it might be more relevant in the long run.
- Use relevant time series and do not use binary values (ones and zeros) to represent occurrences in the sample, if you can avoid it. Rather, use the numerical scale to represent the degree to which it occurred.
- Make sure to measure all the relevant parameters and control for other parameters. This is absolutely necessary in order to be able to draw any conclusions in A/B testing, for example, since you randomize two samples, expose them for the thing you want tested while keeping everything else constant.
- In order to find relevant parameters, you can ask yourself: “What is the main determinator in the consumer’s buying pattern?”. It is a great idea to conduct some proper regression analysis and confirm the main determinator(s).
- Consider the context of the data. For example, be particularly careful when comparing data sets over time and between data samples. Do not automatically assume that the data says a lot about the future - it is a risk to overestimate the predictability in the data.
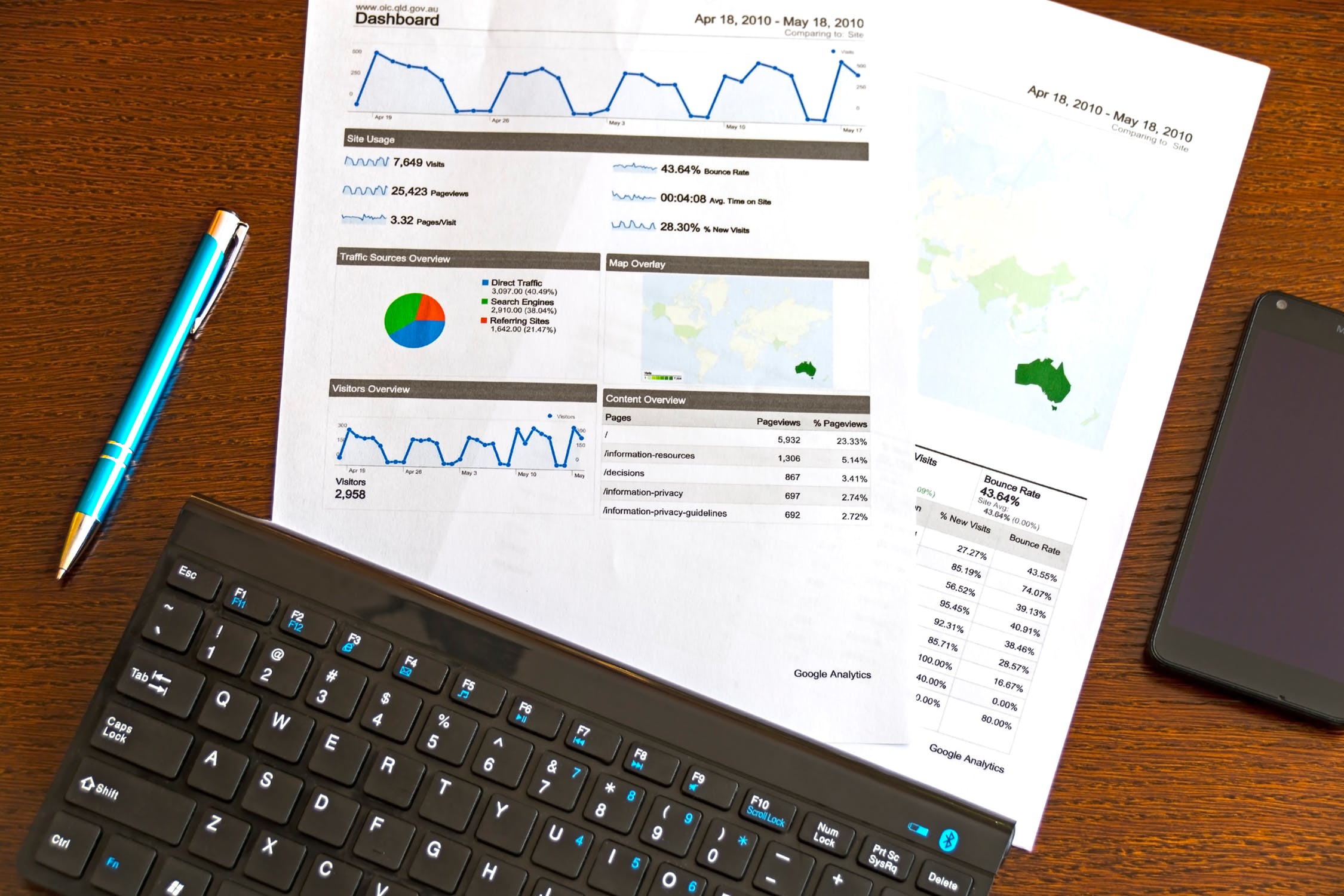
Mathematics
- Beware of, control for and measure the extent to which a trend could be caused by random variation in the sample. Also, make sure that you have statistical significance and enough data.
- A common fault is to assume a linear trendline, but always consider the possibility that it might not be linear. For example, it can be exponential or logarithmic and so on.
- Another common fault is to assume the normal distribution. In order to skip this pitfall, use robust statistics and cover distributions that are not normal as well.
- Data and statistics rarely give a simple Yes or No type of answer. The interpretation must take into account the level of statistical significance. Causation does not imply correlation.
- Always double check with another person without your biases and see what analysis she or he can draw from the data. Also, double check your own analysis from earlier, question your initial hunch and ignore previous results.
- Accept the fact that some data is simply not available and that other data is of terrible quality and should not be used even if it exists. Lots of businesses also collect data for the sake of collecting it and end up measuring unnecessary data. Businesses can absolutely do well without collecting any data - it is a strategic decision, which should consider both the costs and benefits of doing it, just like any other business decision.
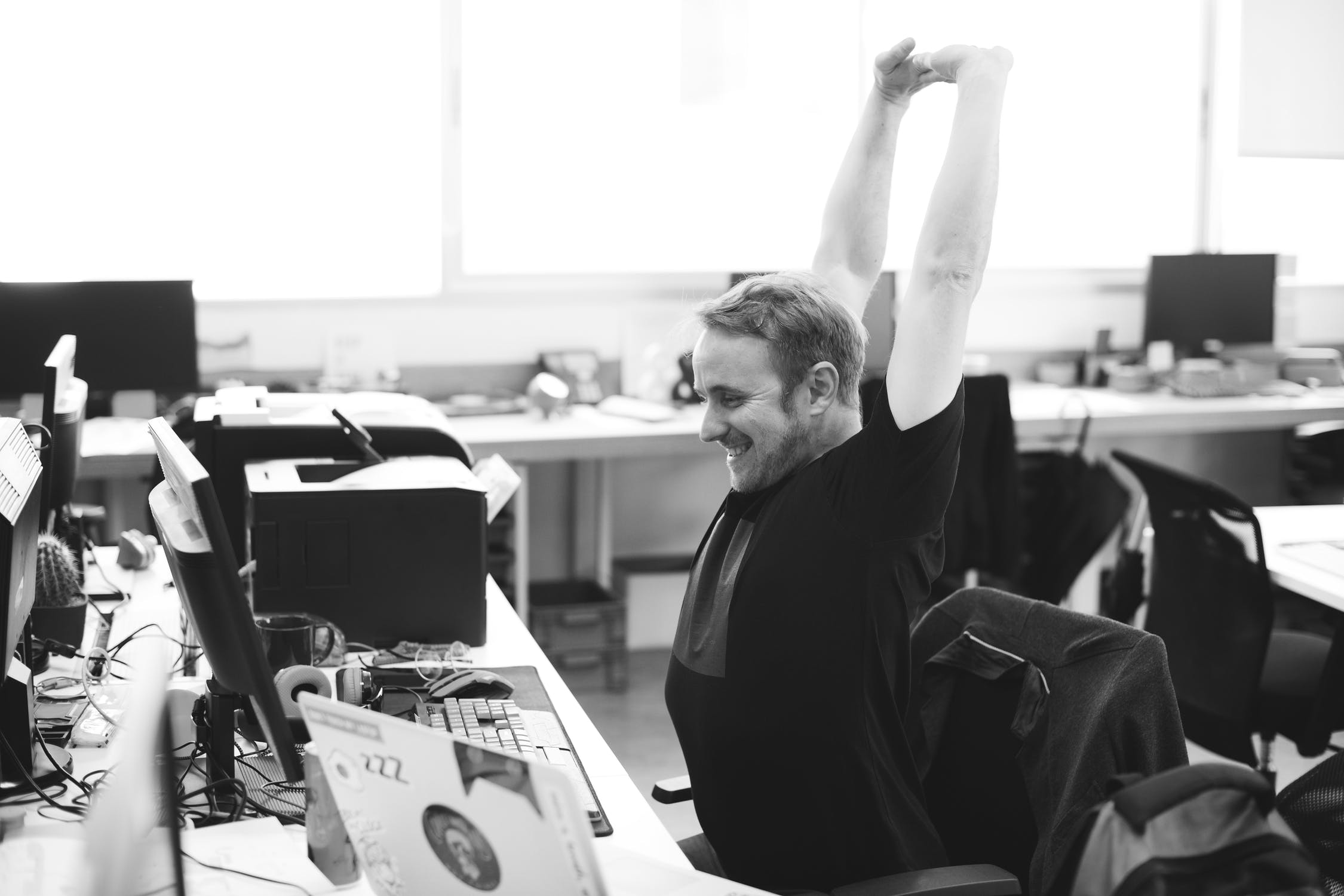
In conclusion, in this article, I have set the stage for the process of implementing data-driven decision making. Lots of businesses want to achieve it and studies have also shown that businesses that use data as a basis for decision making perform better than those which base decisions on feelings or experience. Therefore, I have lined up concrete actions and important concepts to know about when collecting data and there are a lot of challenges and opportunities associated with it. Furthermore, the hype around data is huge and and it probably has not reached its peak yet. Despite the huge hype, businesses do not have to collect data. It is important to start with why, not how, when faced with the strategic decision of designing such a process or not.
Finally, I want to wish everyone best of luck in navigating the huge data jungle out there. :)
/Anna